Does statistics still have a place in the data scientist’s toolbox?
By Kaylea Haynes on October 22, 2018When I first got asked to present at the Royal Statistical Society Leeds and Bradford's 50th anniversary event, with a presentation brief of “talk about using statistical models in data science”, I thought it would be an easy talk to prepare. After all, we use statistics every day as data scientists…don’t we?
I then started to doubt myself when I asked the team how and where they use statistics, and was met by many blank faces. Do we not do statistics?!
When I started to think more in depth about where we use statistics in data science, it became apparent that statistics – or at least the fundamental ideas of statistics – are invaluable tools we do use every single day. But, we either take for granted the statistical origins of the method, or we call it “machine learning.”
Taking the former, particularly in areas such as exploratory data analysis, data cleaning (e.g. imputation) and model evaluations, we take for granted the statistical methods we use, as they have just become part of our everyday data science toolkit. Similarly, we might not rigorously use statistics, such as hypothesis testing, but the knowledge of which helps us to set up unbiased experiments to test business actions.
We also have a bad habit of calling everything machine learning. Take regression, for example; this is originally a statistical method but is very often mistakenly categorised as a machine learning algorithm.
Overall, the key takeaways I got from the event, both in terms of what I learnt during my preparation from my own talk but also from the other presentations from Vinny Davies (School of computing science, University of Glasgow) and Owen Johnson (School of Computing, University of Leeds), are:
- Don’t just use machine learning or AI models because that’s the “trendy” thing to do. If you can use a regression model then do that first before trying something complex – at the very least, it can be a starting point to compare more complex models to.
- But also, statistics has a reputation for being basic, but it can be complex. In fact, in machine learning, it’s generally more important to have methods which are fast to run and work in practice, whereas statistics proves the methods hold asymptotically.
- Don’t take basic statistics for granted – exploratory data analysis is key to building the pathway for more sophisticated models.
- Many machine learning methods are black box, and statistics help us to understand and interpret the models.
- Enthusiasm for using ML within sectors’ IT systems and human generated data dating back many years, such as in the NHS, comes with the risk of unintended consequences which we need to be aware of.
- Statistics are still very useful next to machine learning and AI.
- However, to keep statistics relevant and useful, statisticians need to get better at programming and sharing code online. If a new method is developed in the statistics literature but doesn’t have code available, then it is very unlikely to be used by a data scientist.
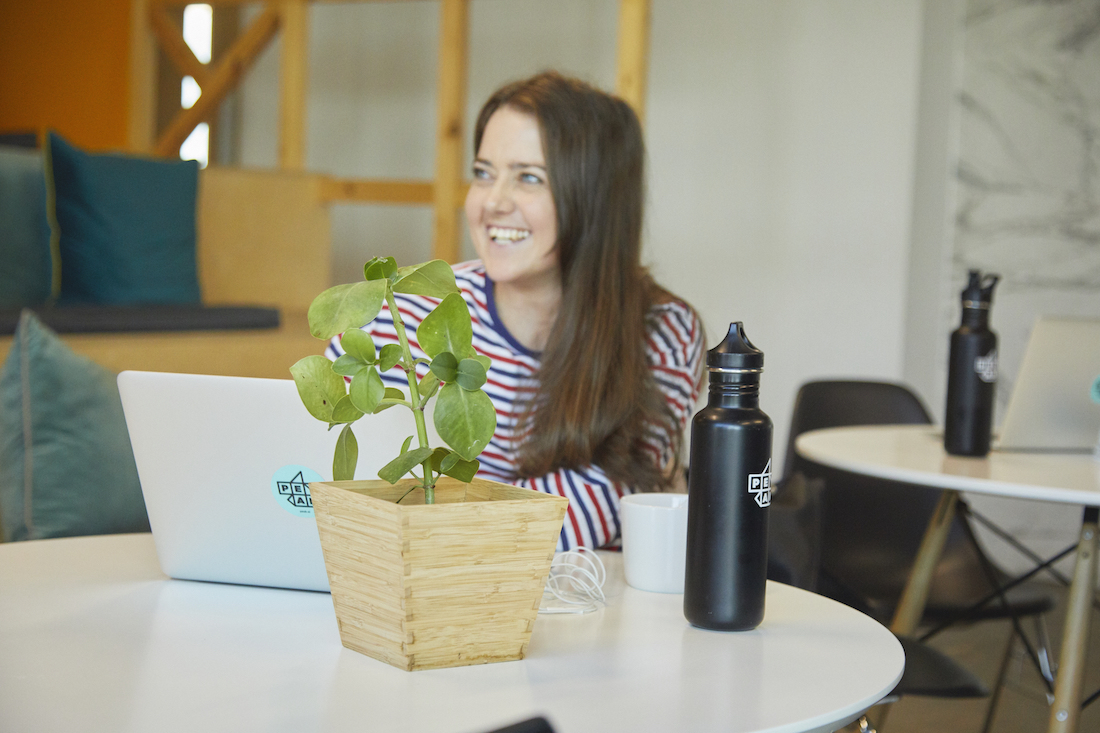
How to get a job in data science
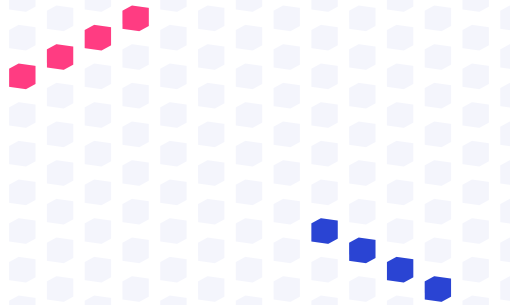
Decision Intelligence: the real new normal
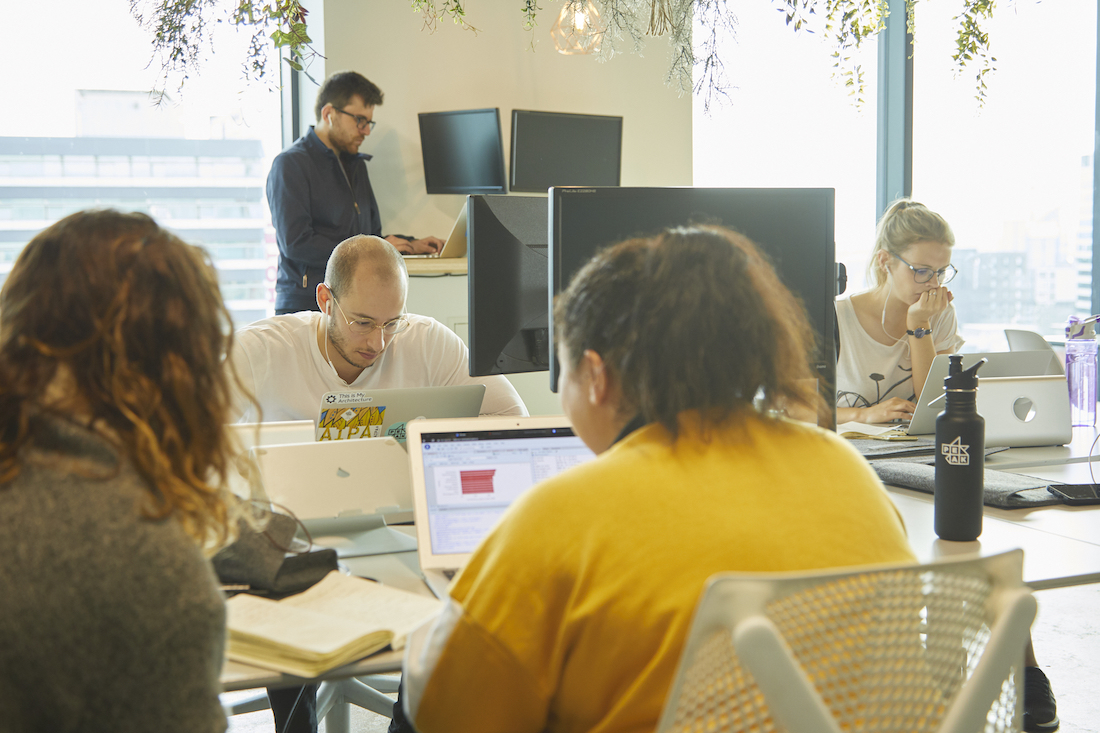