5 examples of AI for retail in practice
By Richard Potter on January 8, 2019The new year is upon us, and retail titles are currently awash with countless articles around predictions and things we can expect for the industry in 2019.
As you might have guessed, artificial intelligence (AI) is enjoying more than its fair share of mentions, and it’s safe to say that big things are expected over the next twelve months from AI. That said, what does AI for retail look like in practice? What sort of things are the successful adopters of these new technologies actually doing, or planning to do?
AI learns from data and in particular, it learns how to predict. These predictions can be used in many applications and then integrated into business systems and processes to automate and truly harness the power of AI. Here are some exciting ways that AI is already being used in the real world…
1. Product recommendations
One of the most powerful applications of AI in retail today is in product recommendations. Personalised product recommendations and content, driven by algorithms, can be embedded in websites, marketing communications and advertising. This goes way beyond simply putting customers into segments and tuning your message – algorithms can learn from the preferences and behaviours of every single customer and personalise the experience for each of them.
You don’t have to look too far for great examples of successful implementations of such systems. Amazon credits 35% of all sales as being driven by its recommendation algorithms, whereas Netflix claims 75% of all content is consumed based on AI-driven recommendations. At Peak, we have seen dramatic increases in customer engagement using these techniques, with our client Footasylum experiencing a 28% uplift in marketing campaign revenues due to personalised product recommendations.
As customers become used to more precise and relevant content and promotions, the companies that are not adopting these techniques will lose business to those who are.
2. Optimising advertising spend
As famous business quotes go, John Wanamaker’s “half of the money I spend on advertising is wasted, the problem is I don’t know which half” is up there with the best. However, those days are numbered (pardon the pun). In a world where we can deeply understand our customers’ preferences and needs, predict their value to us, and model our entire business end-to-end, advertising spend can be optimised using AI to maximise profits and sales.
This requires retailers to combine several different applications of AI and then optimise to the end goal of revenue or profit growth, something that has not been possible without mountains of data and algorithms to do the heavy lifting. However, it works. Lingerie brand Cosabella replaced its ad agency with AI and, after just three months, saw a 336% increase in return on ad spend, also increasing its customer base by 30%.
3. Lead scoring
Lead scoring is a simple, yet powerful, application of the predictive capabilities of AI. For retailers whose sales are made via contact centres, this can augment the capability of the customer care and sales teams to great effect. For example, it allows them to concentrate efforts on customers who are most likely to convert into sales, therefore maximising sales team performance. Companies who sell infrequent but high-value items are most likely to benefit here; car dealerships, gyms and insurers to name but a few.
We’ve seen some fantastic results with our customers in this area. If you’re interested in this aspect of AI, you can read about our work with Regit, which resulted in a 27% increase in revenues from deploying lead scoring techniques.
4. Predicting customer churn and lifetime value
Because of the predictive capabilities of AI, another great application is in looking for early signs that you may be about to lose a particular customer, or that they may churn from your service. Retailers and service providers who charge subscriptions – think gyms, telecoms companies, pay TV operators, apps developers and tech companies – have successfully used churn prediction to enable them to intervene at the right time, with the right offers, to retain their customers. Traditional retailers are now able to do the same thing, triggering retention campaigns and strategies from churn risk scores.
The opposite of this application is predicting the lifetime value or lifetime spend of customers early in their lifecycle with your business. This allows you to identify potential high-value customers early and nurture them to achieve high-value status sooner. You can treat future VIPs as VIPs ahead of time, which is proven to increase loyalty and, ultimately, sales revenues.
5. Propensity and preference scoring
Propensity scoring is the technique of using algorithms to predict an individual’s likelihood to perform a particular action, for example, their propensity to purchase a basket of goods or a specific item. This can be used to determine whether a consumer is in-market or not, too. Preference scoring builds on this and uses the behavioural data of all customers to determine their item and style preferences; for example, that a particular customer is likely to prefer blue sweatshirts from Brand A rather than grey sweatshirts from Brand B.
Both of these techniques can be used to communicate to and target customers who are in the market for particular products at specific times. Akin to product recommendations, market leaders in this area are delivering strong returns from adopting these technologies and strategies.
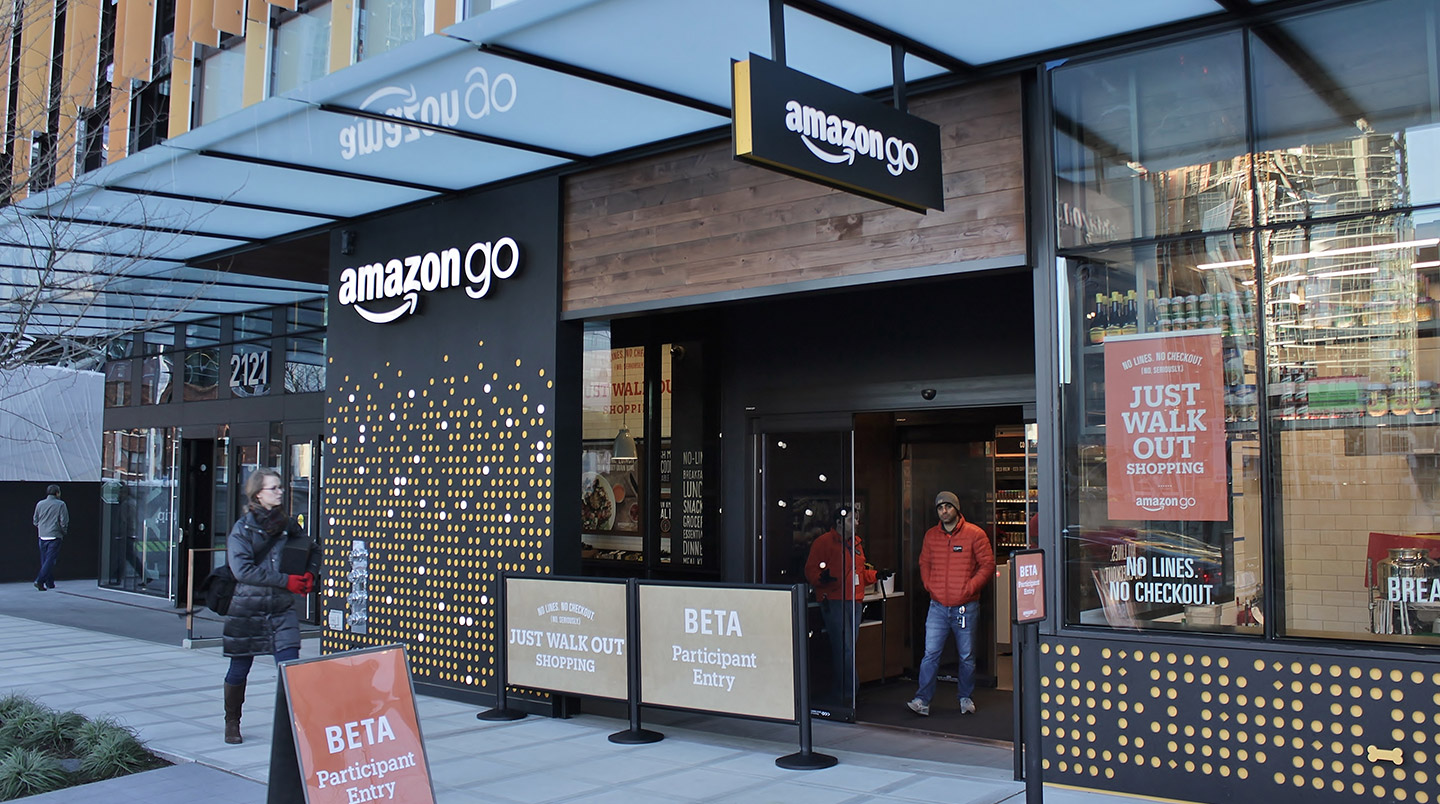
5 (more) examples of AI for retail in practice
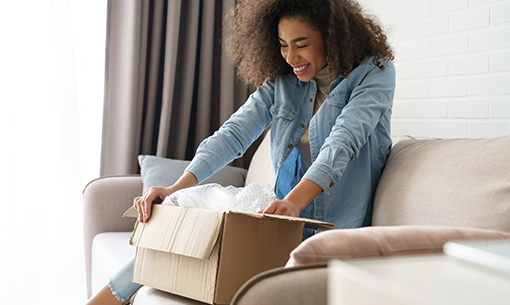